Marketers are always challenged to expand sales beyond “business as usual,” while being good stewards of company resources spent on marketing. Every additional dollar spent on marketing is expected to yield incremental earnings—or else that dollar is better spent elsewhere. You must be able to determine return on advertising spend (ROAS or ROMI, Return on Marketing Investment) for any campaign or platform you add to your marketing mix.
A key driver of positive ROAS is incremental customer actions produced by ad exposure. Confident, accurate measurement of incremental actions is the goal of an effective testing program.
Why do we test campaign performance?
- Because demonstrating incremental actions from a campaign is a victory. You can keep winning by doing more of the same.
- Not finding sufficient incremental actions is an opportunity to reallocate resources and consider new tactics.
- Uncertainty whether the campaign produced incremental actions is frustrating.
- Ending a profitable marketing program because incremental actions were not effectively measured is tragic.
Test for success
When you apply rigorous methods to test the performance of campaigns, you can learn to make incremental improvements in campaign performance. The design of a marketing test requires the following:
- Customer Action to be measured during the test. This action indicates a recognisable step on the path to purchase: awareness, evaluation, inquiry, comparison of offers or products, or a purchase.
- Treatment, i.e., exposure to a brand’s ad during a campaign.
- Prediction regarding the relationship between action and treatment (e.g., Ad exposure produces an increase in purchase likelihood).
- Experimental design is the structure you will create within your marketing campaign to carry out the test.
- Review of results and insights.
Selecting a customer action to measure
Make sure that the customer action you measure in your test is:
- Meaningful to the campaign’s goal. What is the primary goal of the campaign? Is it brand awareness? Web site visits? Inquiries? Completed sales?
- An engagement by the customer. Your measurement should capture meaningful, deliberate interaction of consumers with the brand.
- Attributable to advertising. There should be a reasonable expectation that ad exposure should increase, or perhaps influence the nature of customer actions.
- Abundant in the data. Customer action should be a) plentiful and b) have a high probability of being recorded during the ad campaign (in other words, a high match rate between actions and the audience members).
Selecting campaign treatments
It is best for treatments to be as specific as possible. Ad exposures should be comparable with respect to: brand and offer, messaging, call to action, and format.
Making a prediction
This is the “hypothesis.” Generally, you assume that exposure to advertising will influence customer actions. To do this, you need to reject the conclusion that exposure does NOT affect actions (the “null hypothesis”).
Elements of an experimental design
- An attribution method that links each audience to their purchase action during the test. This consists of a unique identifier of the prospect which can be recognised both in records of the audience and records of the measured action during the measurement period.
- A target audience that receives ad exposure.
- A control audience that does not receive ad exposure. It provides a crucial baseline measurement of action against which the target audience is compared.
- Time boundaries for measurement, related to the treatment:
- Pre-campaign
- Campaign
- Post-campaign
Randomly selected audiences (recommended)
Some audience platforms, such as direct mail and addressable television operators, feature the ability to select distinct audience members in advance. Randomly selected audiences can generally be assumed to be similar in all respects except ad exposure.
The lift of the action rate is simple to calculate:
Campaign Lift = (Action Rate (target) / Action Rate (control)) -1
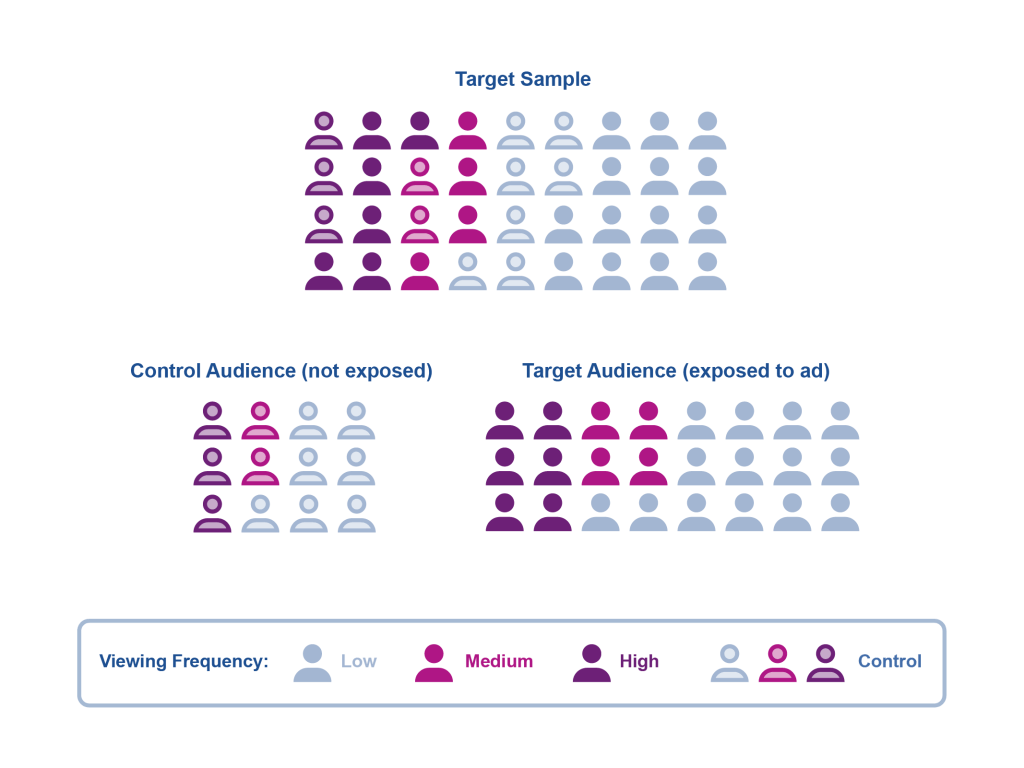
Non-randomly selected audiences are more difficult, but still possible to measure effectively. There may be inherent biases between them that may or may not be obvious.
To measure campaign performance, we must first account for any pre-existing differences in customer actions, and then adjust for these when measuring the effect of ad exposure.
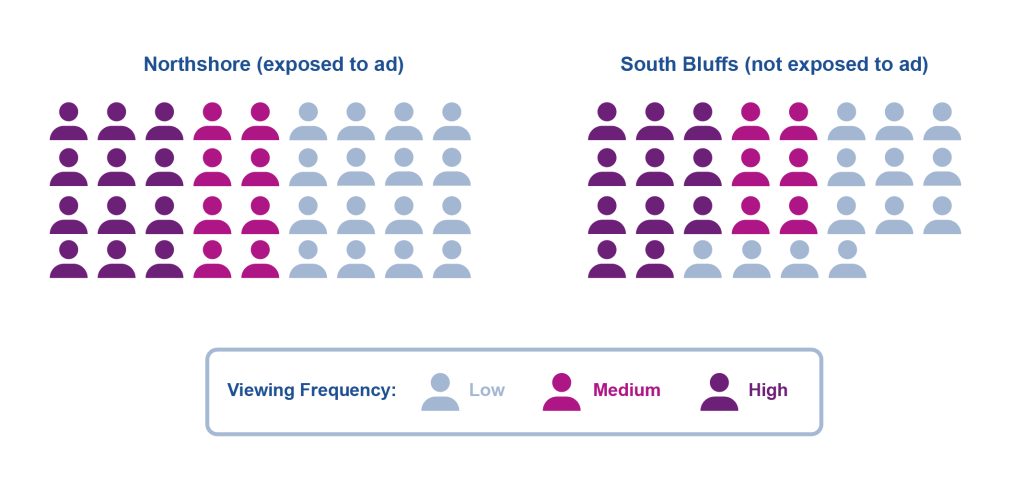
Typically, the pre-campaign period (and possibly the post-campaign period as well) are used to obtain a baseline comparison of actions between the two audiences. This is a “difference of differences” measurement:
Baseline lift = (Action Rate (target) / Action Rate (control)) -1
Campaign Lift = (Action Rate (target) / Action Rate (control)) -1
Net campaign lift (advertising effect) = Campaign Lift – Baseline Lift
Analysing results and insights
- How large is the lift? This is generally expressed as a percentage increase in action rate for the target audience vs. the control audience.
- Are we confident that the lift is real, and not just random noise in the data? This question is answered with the “confidence level.”. 95% confidence means the probability of a “true positive” result is 95%; and the probability of a “false positive” due to random error is 5%.
- What was the campaign cost per incremental action? If you also know the expected revenue from each incremental action, you can project out incremental revenue, from which you can calculate return on ad spend.
- Other insights: Do the results make directional sense (we would hope that ad exposure will cause an increase in customer actions, not a decrease)? Does action rate generally increase with the number of ad exposures?
Summary
Well-designed testing and measurement practices allow you to learn from individual advertising campaigns to improve decision-making. The ability to draw confident conclusions from campaigns will allow experimentation with different strategies, tactics, and communication channels to maximise performance. These test-and-learn strategies also enhance your ability to adjust to marketplace trends by monitoring campaign performance.